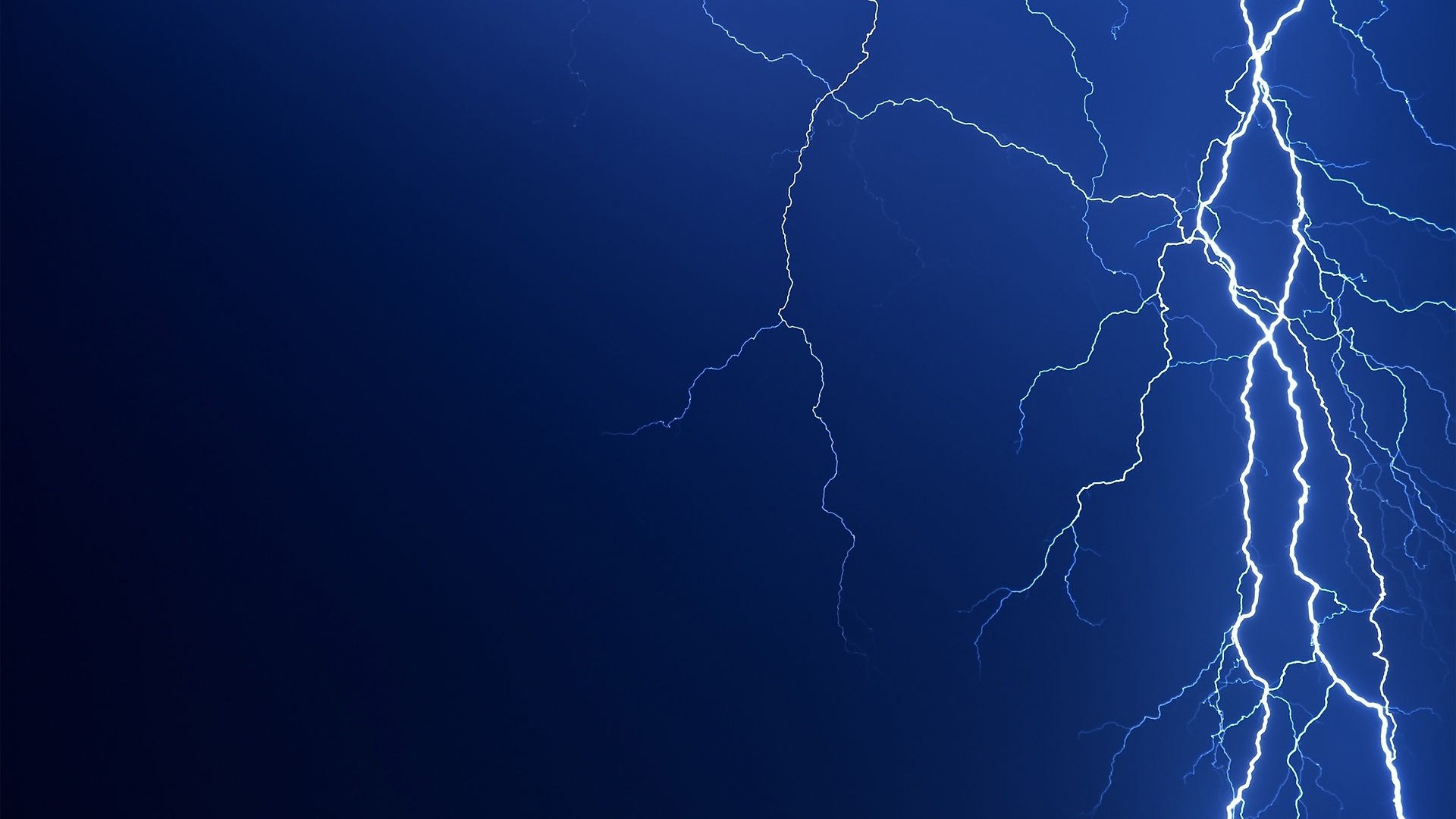
EN
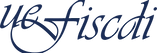
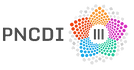
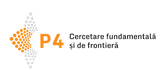
2024
-
Intl. Roadmap Devices & Systems (IRDS™), 2023. https://irds.ieee.org/editions/2023
-
W. Cao et al., The future transistors, Nature, vol. 620, no. 7974, pp. 501-515, 17 Aug. 2023. https://doi.org/10.1038/s41586-023-06145-x
-
G. Finocchio et al., Roadmap for unconventional computing with nanotechnology, Nano Futures, vol. 8, art. 012001 (pp. 1-59), 28 Mar. 2024. https://doi.org/10.1088/2399-1984/ad299a
-
OpenROAD, ASAP7 7nm predictive PDK, 15 Dec. 2020. https://github.com/The-OpenROAD-Project/asap7
-
Arizona State University, ASAP7/ASU 7nm predictive PDK, Feb. 2021. https://asap.asu.edu/
-
L.T. Clark et al., ASAP7: A 7-nm FinFET predictive process design kit, Microelectr. J., vol. 53, pp. 105-115, Jul. 2016. https://doi.org/10.1016/j.mejo.2016.04.006
-
M. Choi et al., Modeling performance and thermal induced reliability issues of a 3nm FinFET logic chip operation in a fan-out and a flip-chip packages, Proc. Intl. Conf. Thermal & Thermomech. Phenom. Electr. Syst. (ITherm), Las Vegas, NV, USA, pp. 107-112, 28-31 May 2019. https://doi.org/10.1109/ITHERM.2019.8757442
-
Y. Lee et al., Investigation on the effects of interconnect RC in 3nm technology node using path-finding process design kit, IEEE Access, vol. 10, pp. 80695-80702, Aug. 2022. https://doi.org/10.1109/ACCESS.2022.3195506
-
J. Jeong et al., Design technology co-optimization and time-efficient verification for enhanced pin accessibility in the post-3 nm node, IEEE Access, vol. 12, pp. 97557-97571, Jul. 2024. https://doi.org/10.1109/ACCESS.2024.3427332
-
G. Sisto et al., Evaluation of nanosheet and forksheet width modulation for digital IC design in the sub-3nm era, IEEE Trans. VLSI Syst., vol. 30, no. 10, pp. 1497-1506, Oct. 2022. https://doi.org/10.1109/TVLSI.2022.3190080
-
M. Hashemipour et al., 21T ternary full adder based on capacitive threshold logic and carbon nanotube FETs, IEEE Trans. Nanotech., vol. 23, pp. 338-345, Apr. 2024. https://doi.org/10.1109/TNANO.2024.3386825
-
N. Safron et al., High performance transistor of aligned carbon nanotubes in a nanosheet structure, IEEE Symp. VLSI Circ. & Syst. (VLSI), Honolulu, HI, USA, 16-20 Jun. 2024. https://vlsi24.mapyourshow.com/mys_shared/vlsi24/handouts/T3-3_GregPitner.pdf
-
J. Si et al., A carbon-nanotube-based tensor processing unit, Nature Electr., vol. 7, no. 8, pp. 684-693, Aug. 2024. https://doi.org/10.1038/s41928-024-01211-2
-
R.-M. Beiu, Theoretical and experimental contributions to a new class of sensors for assessing mechanical deformations, PhD thesis, “Politehnica” University of Bucharest, Bucharest, Romania, Nov. 2007. 681.586.5:539.37(043), http://romdoc.upb.ro/record/518
-
R.M. Beiu et al., Fiber optic mechanical deformation sensors employing perpendicular photonic crystals, Optics Express, vol. 25, no. 19, pp. 23388-23398, Sep. 2017. https://doi.org/10.1364/OE.25.023388
-
C.E. Shannon, A symbolic analysis of relay and switching circuits, Trans. AIEE, vol. 57, no. 12, pp. 713-723, Dec. 1938. https://doi.org/10.1109/T-AIEE.1938.5057767 [see also https://hdl.handle.net/1721.1/11173]
-
V. Jandieri et al., Functional all-optical logic gates for true time-domain signal processing in nonlinear photonic crystal waveguides, Optics Express, vol. 28, no. 12, art. 18317 (pp. 1-15), 8 Jun. 2020. https://doi.org/10.1364/OE.395015
-
L.P. Caballero et al., Photonic crystal integrated logic gates and circuits, Optics Express, vol. 30, no. 2, art. 1976 (pp. 1-18), 17 Jan. 2022. https://doi.org/10.1364/OE.444714
-
Y. Huang et al., Design of multifunctional all-optical logic gates based on photonic crystal waveguides, Appl. Optics, vol. 62, no. 3, pp. 774-781, 20 Jan. 2023. https://doi.org/10.1364/AO.473410
-
H. Chen et al., Simple and reconfigurable all-optical logic gate, Optics & Laser Tech., vol. 170, art. 110297 (pp. 1-6), Mar. 2024. https://doi.org/10.1016/j.optlastec.2023.110297
-
Y. Shen et al., Deep learning with coherent nanophotonic circuits, Nature Photonics, vol. 11, no. 7, pp. 441-446, Jul. 2017. https://doi.org/10.1038/nphoton.2017.93
-
T.W. Hughes et al., Training of photonic neural networks through in situ backpropagation and gradient measurement, Optica, vol. 5, no. 7, pp. 864-871, Jul. 2018. https://doi.org/10.1364/OPTICA.5.000864
-
S. Pai et al., Experimentally realized in situ backpropagation for deep learning in photonic neural networks, Science, vol. 380, no. 6643, pp. 398-404, 28 Apr. 2023. https://doi.org/10.1126/science.ade8450
-
B. Wu et al., Real-valued optical matrix computing with simplified MZI mesh, Intell. Comput., vol. 2, art. 0047 (pp. 1-8), Sep. 2023. https://doi.org/10.34133/icomputing.0047
-
K. Yao et al., Intelligent nanophotonics: Merging photonics and artificial intelligence at the nanoscale (Review), Nanophotonics, vol. 8, no. 3, pp. 339-366, Jan. 2019. https://doi.org/10.1515/nanoph-2018-0183
-
F.P. Sunny et al., A survey on silicon photonics for deep learning, ACM J. Emerg. Technol. Comp. Syst., vol. 17, no. 4, art. 61 (pp. 1-57), Jun. 2021. https://doi.org/10.1145/3459009
-
N. Peserico et al., Integrated photonic tensor processing unit for a matrix multiply: A review, J. Lightwave Technol., vol. 41, no. 12, pp. 3704-3716, 15 Jun. 2023. https://doi.org/10.1109/JLT.2023.3269957
-
B. Romeira et al., Brain-inspired nanophotonic spike computing: challenges and prospects, Neuromorph. Comput. Eng., vol. 3, art. 033001 (pp. 1-32), 14 Jul. 2023. https://doi.org/10.1088/2634-4386/acdf17
-
N. Stroev and N.G. Berloff, Analog photonics computing for information processing, inference, and optimization (Review), Adv. Quantum Technol., vol. 6, no. 9, art. 2300055 (pp. 1-42), Sep. 2023. https://doi.org/10.1002/qute.202300055
-
S. Ou et al., Hypermultiplexed integrated tensor optical processor, Tech. Rep., arXiv:2401.18050 [cs.ET], 13 Feb. 2024. https://doi.org/10.48550/arXiv.2401.18050
-
Z. Hu et al., Large-scale photonic chiplet Taichi empowers 160-TOPS/W artificial general intelligence, Science, vol. 384, no. 6692, pp. 202-209, 12 Apr. 2024. https://doi.org/10.1126/science.adl1203
-
C. Ting-Fang and L. Ly, TSMC aims to ready next-gen silicon photonics for AI in 5 years, Nikkei Asia, 3 Sep. 2024. https://asia.nikkei.com/Business/Technology/TSMC-aims-to-ready-next-gen-silicon-photonics-for-AI-in-5-years
-
A. Noy and S.B. Darling, Nanofluidics makes a splash, Science, vol. 379, no. 6628, pp. 143-144, 12 Jan. 2023. https://doi.org/10.1126/science.adf6400
-
P. Robin and L. Bocquet, Nanofluidics at the crossroads, J. Chem. Phys., vol. 158, nr. 16, art. 160901 (pp. 1-14), 28 Apr. 2023. https://doi.org/10.1063/5.0143222
-
A.P. Singh et al., Logic operations in fluidics as foundation for embedded biohybrid computation (Review), Device, vol. 1, no. 6, art. 100220 (pp. 1-19), 22 Dec. 2023. https://doi.org/10.1016/j.device.2023.100220
-
L. Yu et al., Bioinspired nanofluidic iontronics for brain-like computing (Review), Nano Res., vol. 17, no. 2, pp. 503-514, Feb. 2024. https://doi.org/10.1007/s12274-023-5900-y
-
M. Huang et al., Electrochemical ionic synapses: Progress and perspectives (Review), Adv. Mater., Sp. Iss. Neuromorphic Engineering: From Materials to Device Application, vol. 35, no. 37, art. 2205169 (pp. 1-33), 14 Sep. 2023. https://doi.org/10.1002/adma.202205169
-
W. Liu et al., Bioinspired carbon nanotube-based nanofluidic ionic transistor with ultrahigh switching capabilities for logic circuits, Sci. Adv., vol. 10, no. 11, art. eadj7867 (pp. 1-8), 13 Mar. 2024. https://doi.org/10.1126/sciadv.adj7867
-
P. Robin et al, Modeling of emergent memory and voltage spiking in ionic transport through angstrom-scale slits, Science, vol. 373, no. 6555, pp. 687-691, 6 Aug. 2021. https://doi.org/10.1126/science.abf7923
-
M. Gao et al., A nanoconfined water-ion coordination network for flexible energy-dissipation devices, Adv. Mater., vol. 35, no. 42, art. 2303759 (pp. 1-11), 19 Oct. 2023. https://doi.org/10.1002/adma.202303759
-
G. Paulo et al., Hydrophobically gated memristive nanopores for neuromorphic applications, Nature Comm., vol. 14, art. 8390 (pp. 1-9), 18 Dec. 2023. https://doi.org/10.1038/s41467-023-44019-y
-
G. Papandroulidakis et al., Practical implementation of memristor-based threshold logic gates, IEEE Trans. Circ. & Syst. I, vol. 66, no. 8, pp. 3041-3051, Aug. 2019. https://doi.org/10.1109/TCSI.2019.2902475
-
E.J. Fuller et al., Li-ion synaptic transistor for low power analog computing, Adv. Mater., vol. 29, no. 4, art. 1604310, 25 Jan. 2017. https://doi.org/10.1002/adma.201604310
-
N.A. Nguyen, Lithium-based nano-ionic synaptic transistors for neuromorphic computing, PhD thesis, Univ. Paris-Saclay, 19 Sep. 2022. https://theses.hal.science/tel-04213026